As businesses aim to deliver consistent, personalized engagement across multiple channels, a brand LLM stands out as a transformative solution.
Powered by generative AI, a brand LLM ensures that customer interactions remain consistent, compliant and personalized at scale, effectively shaping every touchpoint to reflect the company’s identity.
What is a brand LLM?
A brand LLM (large language model) is a generative AI-powered system fine-tuned to embody a company’s brand identity, values, guidelines and content standards.
It acts as a centralized, dynamic resource for creating, managing and delivering consistent and personalized brand content across all customer interactions and engagement channels.
3 reasons why your company needs a brand LLM
Modern brands must balance consistency, compliance and personalization to stay competitive. Traditional methods of managing brand assets no longer meet these demands. A brand LLM addresses these challenges in three critical ways.
1. It becomes your company’s most valuable asset
A brand LLM encapsulates everything your company brand represents, forming the foundation for every customer interaction. Up to 90% of S&P companies’ value is tied to intangible assets like “future customer intentions to buy,” as opposed to tangible assets like buildings and inventory.
Managing your brand goes beyond merely storing assets on a shared server. It’s about shaping preferences in customers’ minds and hearts.
2. It ensures compliance and consistency across markets
A brand LLM ensures every interaction aligns with local laws, product variations and brand guidelines. For instance:
- Displaying a car door knob color on an ad that isn’t for sale in a country hinders sales.
- A tagline that isn’t translated into French can lead to a lawsuit.
A brand LLM automates compliance and ensures consistency across all markets.
3. It unlocks unprecedented personalization
A brand LLM enables unprecedented personalization by making real-time customization of copy, images and videos possible. While text personalization is now common in campaigns, visuals often remain static. The potential for fully personalized visuals at scale is still untapped. A brand LLM also helps navigate the fine line of hyper-personalization, ensuring it avoids the “creepy factor” by knowing when not to personalize.
While the benefits of a brand LLM are clear, realizing its full potential requires integrating it seamlessly into your existing infrastructure. This is where the connection between the data and content layers becomes critical.
When the data layer meets the content layer
Many brands excel at collecting and interpreting customer data but still struggle with poor content delivery. Most brands have a data layer that efficiently collects, processes and streams audience insights into engagement channels.
In contrast, the content layer, which handles customer-facing elements like copy, images, and videos, remains siloed, fragmented and manual. While the data layer is nearly seamless, the content layer faces significant integration challenges.
This gap is especially evident in time-sensitive campaigns across complex markets with multiple segments, languages, and currencies. For example:
- A delayed product image can cost a tech manufacturer millions in lost revenue and digital shelf space.
- A real-time, hyper-personalized in-basket offer is crucial for keeping customers engaged in an ecommerce environment.
Why the content layer is broken
The content layer is often non-existent or, at best, broken. While the data layer seamlessly processes information through tightly integrated steps using APIs and (reverse) ETL mechanisms, the same level of integration is lacking in the content supply chain.
The systems and elements in the content layer are either not integrated or poorly integrated.
- Corporate identity: Guidelines for house style and brand identity.
- Creative design software: Design software for asset creation.
- Digital asset management (DAM): Storage for brand and campaign media
- Web2Publish (W2P): Templatized collateral production.
- Product information management (PIM).
- Content management systems (CMS).
- Digital eXperience platforms (DXP).
- Data management (MDM): Corporate entity definitions.
- Engagement channels: Email, push, SMS, chat, in-app and more.
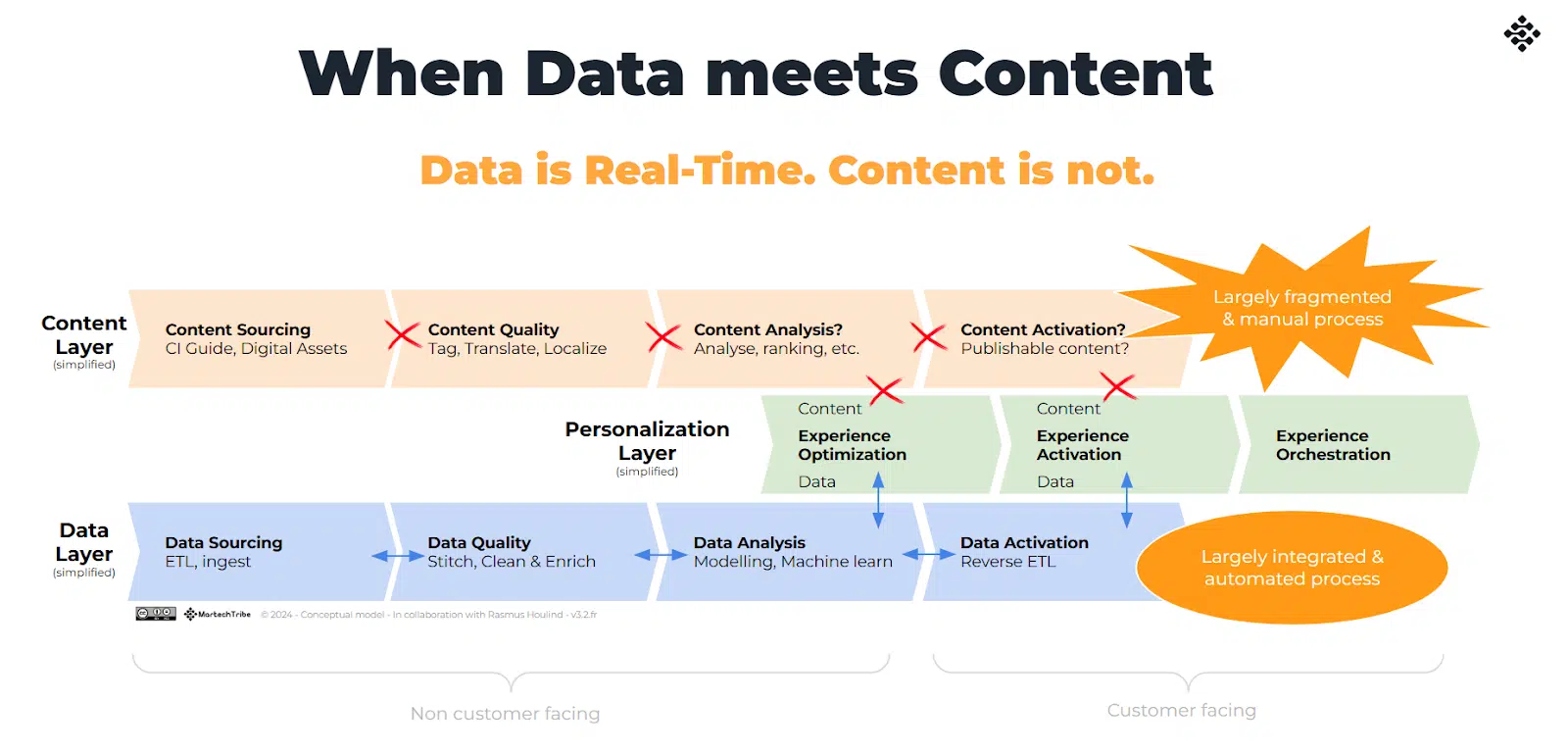
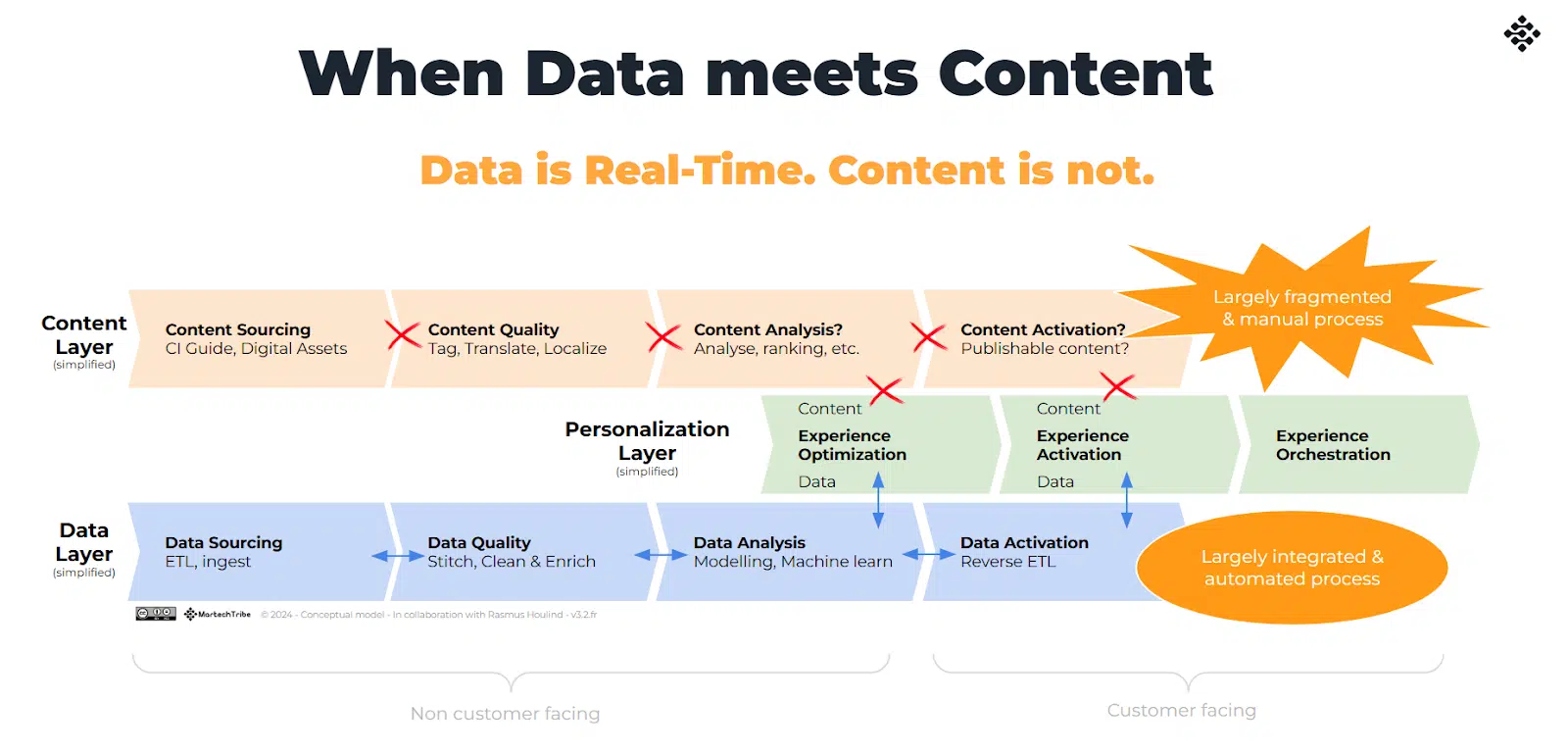
The challenge isn’t due to content vendors doing a poor job. Instead, rendering content in real-time is complex due to three major obstacles.
- Creativity: Human creativity is hard to mimic for computers, hence the laborious manual work. (Consider internal studios.)
- Computing power: Rendering large files, such as image and video assets, requires significantly more processing power than numeric data. (Think about the size of Photoshop, MOV or MP4 files.)
- Integration: Seamlessly transferring large content files across systems is still a manual process. (Think WeTransfer or WeSendIt.)
These challenges have left the content layer immature for decades, making it difficult to realize its full potential — until now.
Generative AI changes the game, providing the creativity, computational power and integration needed to revolutionize the content layer.
How to build a content layer with generative AI
To shape their content layer, brands should start by understanding the importance of the master file.
The master file is the original, highest-quality version of a digital asset — uncompressed, high-resolution and complete with metadata (EXIF, IPTC, XMP). It serves as the source for creating derivative versions for web, print or social media.
However, regional adaptations often dilute the brand and message, which has led to the long-standing best practice of restricting master file access to trained creatives in studios.
“Don’t share the master file.”
– John van Tuyll, Global Brand Marketing Operations, Adidas
Now, with generative AI, the concept of the master file can evolve. Instead of using static master files, brands can create a brand LLM that serves as the dynamic source for content creation.
There are multiple brand LLM use cases in daily marketing activities. Maybe the brand LLM is used in its own user interface where content can be uploaded or accessed via an API to render a specific output. The output could look like this.
- Annotating brand materials to ensure compliance and consistency.
- Suggesting adaptations to boost conversion rates or meet local regulations (a use case recently launched by Jasper.ai).
- Optimizing brand materials for data-driven, high-performance campaigns.
- Producing real-time content for collateral and campaigns.
- Activating channel-specific content tailored to audience parameters and streamed directly via engagement solutions to customers.
Dig deeper: The opportunities for AI in digital asset management
How to create brand master LLMs
Brands can build a brand LLM by integrating customer and brand data into AI systems. This can be achieved in four ways.
- Fine-tuning pre-trained models: Customize existing LLMs with your brand’s content.
- Prompt engineering: Create prompts to align AI outputs with your brand’s tone.
- Embedding custom data: Include resources like product catalogs or FAQs.
- API integration: Use APIs like OpenAI’s GPT to embed LLM functionality into workflows.
These methods can work together in a retrieval-augmented generation (RAG) framework, which retrieves relevant data, applies prompts and generates brand-specific outputs. This approach ensures real-time adaptability for applications like customer support or campaign management.
“Generating new content directly with an LLM delivered significantly better results compared to starting with derivative assets.”
– Rasmus Houlind, Agilic
By managing content using a brand LLM, companies can prioritize and streamline their existing content, producing relevant messages at the right time and place.
What your brand LLM should cover
When fine-tuning your Brand LLM, include the following levels:
Brand level
Define your brand identity and reputation, using guidelines or positioning it as a relatable personality. Customers connect emotionally with brands, often viewing them as extensions of their founders’ intentions.
Campaign level
Outline (single-minded) value propositions, messaging, tone and media mix strategies. Campaigns bridge brand identity with actionable tactics to influence customer attitudes and behaviors.
Collateral level
Specify prompts for assets like product shots, logos and taglines. This level ensures consistency and evaluates effectiveness through return-on-content metrics.
Content level
Address co-branded materials, third-party collaborations and snackable content for digital platforms. This ensures your brand scales seamlessly across various use cases.
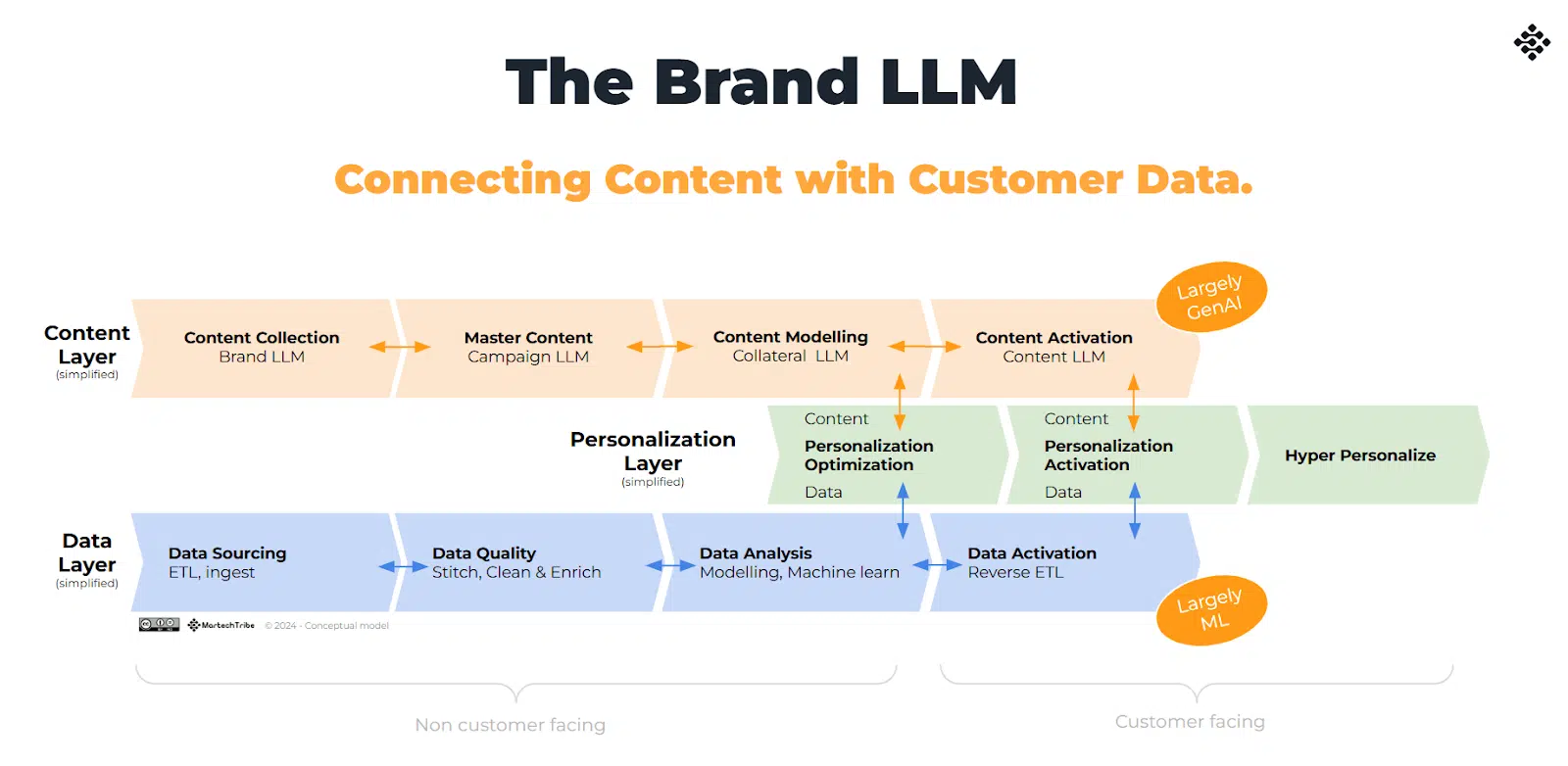
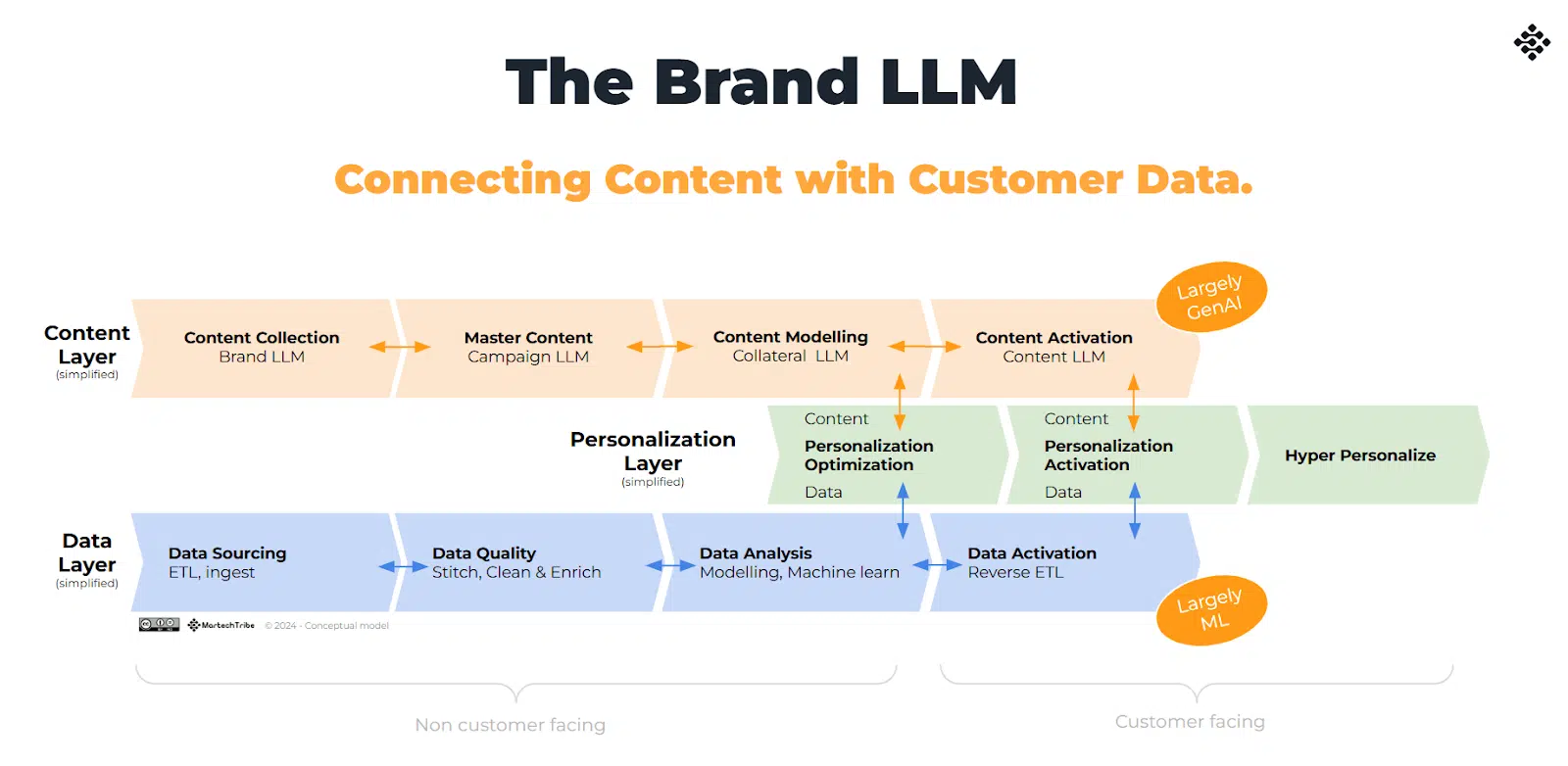
By tackling these levels, a brand LLM evolves into a structured, comprehensive solution for real-time, integrated cross-channel content creation. This unlocks the previously untapped competitive advantage of delivering the right content at the right time to the right person. What is “right” is now defined by insights from the data layer, combined with generative AI’s analysis of the highest return on content.
Dig deeper: A co-pilot approach to genAI (with prompt examples)
Contributing authors are invited to create content for MarTech and are chosen for their expertise and contribution to the martech community. Our contributors work under the oversight of the editorial staff and contributions are checked for quality and relevance to our readers. The opinions they express are their own.